So, let me go back to cybersecurity. Sorry for the long, boring mathematical-based explanation in the last part, but if one wants to use a given tool, he/she must understand how it works and, more essentially, its limits. And I shall give you my list of concerns why believing in the current cybersecurity hype can be dangerous for you and your organization:
- We must not compare a Machine Learning model to the human brain: We have no idea how the human brain works, and more especially how ideas creation and generalization work. Additionally, the pure power consumption of a machine learning model is times bigger than a human brain. Sure it is faster but much more expensive. The average power consumption of a typical adult is 100 Watts, and the brain consumes 20% of this, making the brain’s power consumption around 20 W. For comparison, Google’s DeepMind project uses a whole data center to achieve the same result, which a two-year-old kid does with 20 W.
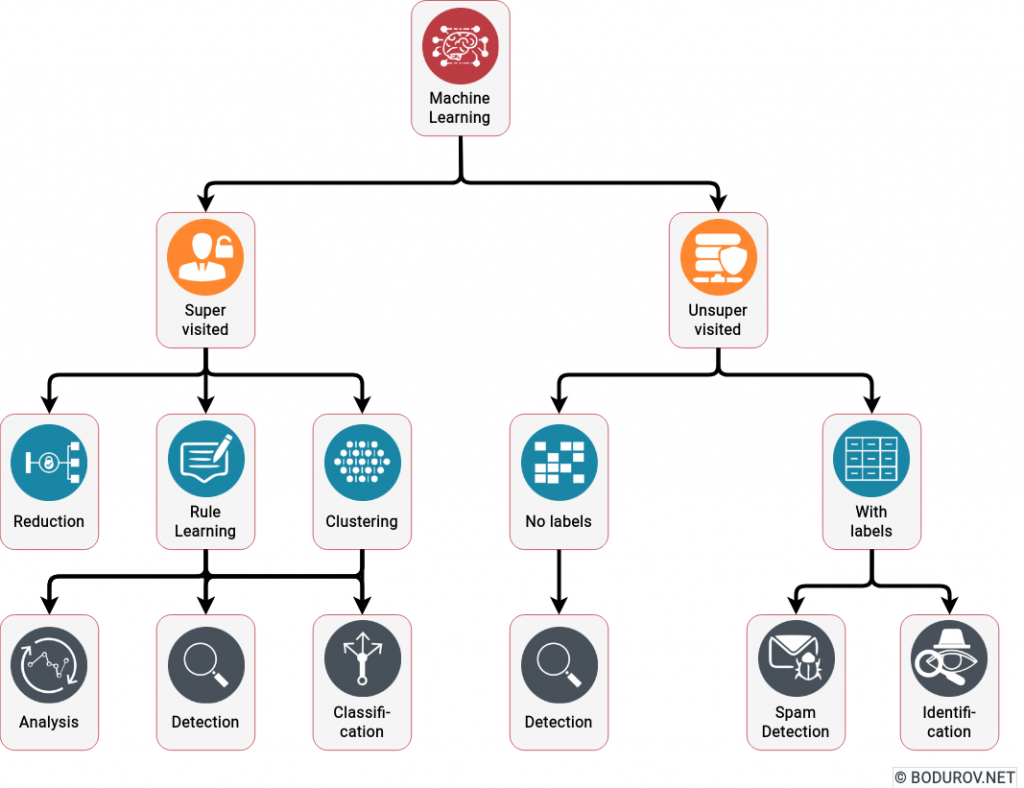
- Machine learning is weak in generalization: The primary purpose of polynomial generation is to solve the so-named categorization problem. We have a set of objects with characteristics, and we want to put them in different categories. Machine learning is good at that. However, if we add a new category or dramatically change the set of objects, it fails miserably. In comparison, the human brain is excellent in generalization or, in social words – improvisation. If we transfer this to cybersecurity – ML is good in detection, but weak in prevention.
- Machine Learning offers nothing new in Cybersecurity: For a long time, antivirus and anti-spam software have used rule engines to categorize whether the incoming file or email is malicious or not. Essentially, this method is just a simple categorization, where we mark the incoming data as harmful or not. All of the currently advertised AI-based cybersecurity platforms do that – instead of making the rule engine manually, they use Machine Learning to train their detection abilities.
In conclusion, cybersecurity Machine Learning models are good in detection but not in prevention. Marketing them as the panacea for all your cybersecurity problems could be harmful for organizations. A much better presentation of these methods is to call them another tool in the cybersecurity suite and use them appropriately. A good cybersecurity awareness course will undoubtedly increase your chances of prevention rather than the current level of Artificial Intelligence systems.